기세환 박사과정 논문 IEEE ACCESS 저널 게재 승인
기세환 박사과정생의 논문이 IEEE ACCESS 저널에 accept되었습니다. 축하합니다!
Title: Learning-based JND-directed HDR Video Preprocessing for Perceptually Lossless Compression with HEVC
Authors: Sehwan Ki and Munchurl Kim
Abstract:
The final consumer of videos is mostly human. Therefore, if videos can be compressed by fully utilizing the perception characteristics of human visual systems (HVS), the bitrates of the compressed videos can be significantly reduced with subjective visual quality degradation as little as possible. Based on this, we newly propose a learning-based Just Noticeable Distortion (JND)-directed preprocessing scheme for perceptual video compression, especially for 10-bit High Dynamic Range (HDR) videos, which is called the HDR-JNDNet. Our HDR-JNDNet effectively suppresses the perceptual redundancy of 10-bit HDR video signals so that the compression efficiency can be significantly enhanced for the HEVC main10 profile encoder. To our best knowledge, our work is the first approach to training a CNN-based model to directly generate the JND-directed suppressed frames of 10-bit HDR video with the negligible perceptual quality difference between the decoded frames for the original HDR video input with and without the preprocessing by our HDR-JNDNet. Via intensive experiments, when the HDR-JNDNet is applied as preprocessing for the HDR video input before compression, it allows to remarkably save the required bitrates up to the maximum (average) 40.66% (18.37%) for 4K-UHD/HDR test videos, with little subjective video quality degradation without increasing the computational complexity.
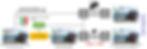